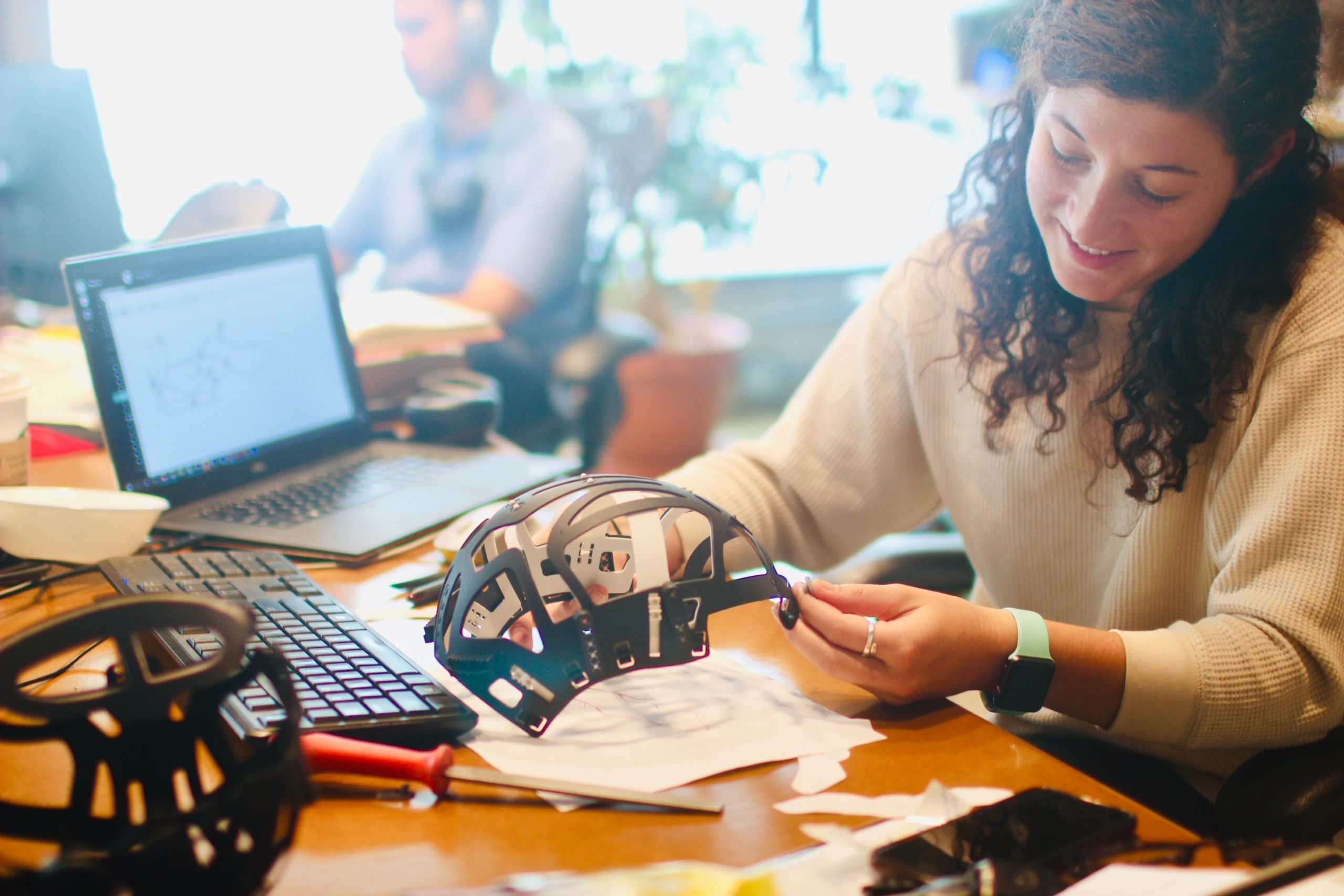
Over the past few years, I’ve been a mentor for startups launching from Washington State University (Go, Cougs!). Most of the co-founders I work with struggle to understand their product-market fit. They aren’t alone – customer discovery is a continuing challenge even for experienced founders. It’s easy to lose sight of crucial variables or over-emphasize trivial characteristics. In this article, I explain a systematic approach to building a model, where to find data, and how to incorporate the analysis in a cyclical process. I’ve also included three tips to help you with frequent problems. Please give this a try, and let me know your outcomes!
At its simplest, data science is the extraction of knowledge from data. Businesses of all sizes need this knowledge, but it’s key to startup success. Even science -or technology-focused founders struggle with acquiring and evaluating data to discover their customers or refine a product or service. The good news is you don’t need to be a data scientist, nor do you need expensive computer tools to assemble and analyze a set of business data for insight relevant to your business. A thoughtful process and a small dataset are all you need. Too much data, even if applicable, can obscure the principal messages or make fringe points seem more decisive. In this article, I will share a systematic process for getting started with a model, finding relevant data, and using the results to improve the model. I also share three tips to overcome pitfalls you may face during the process.
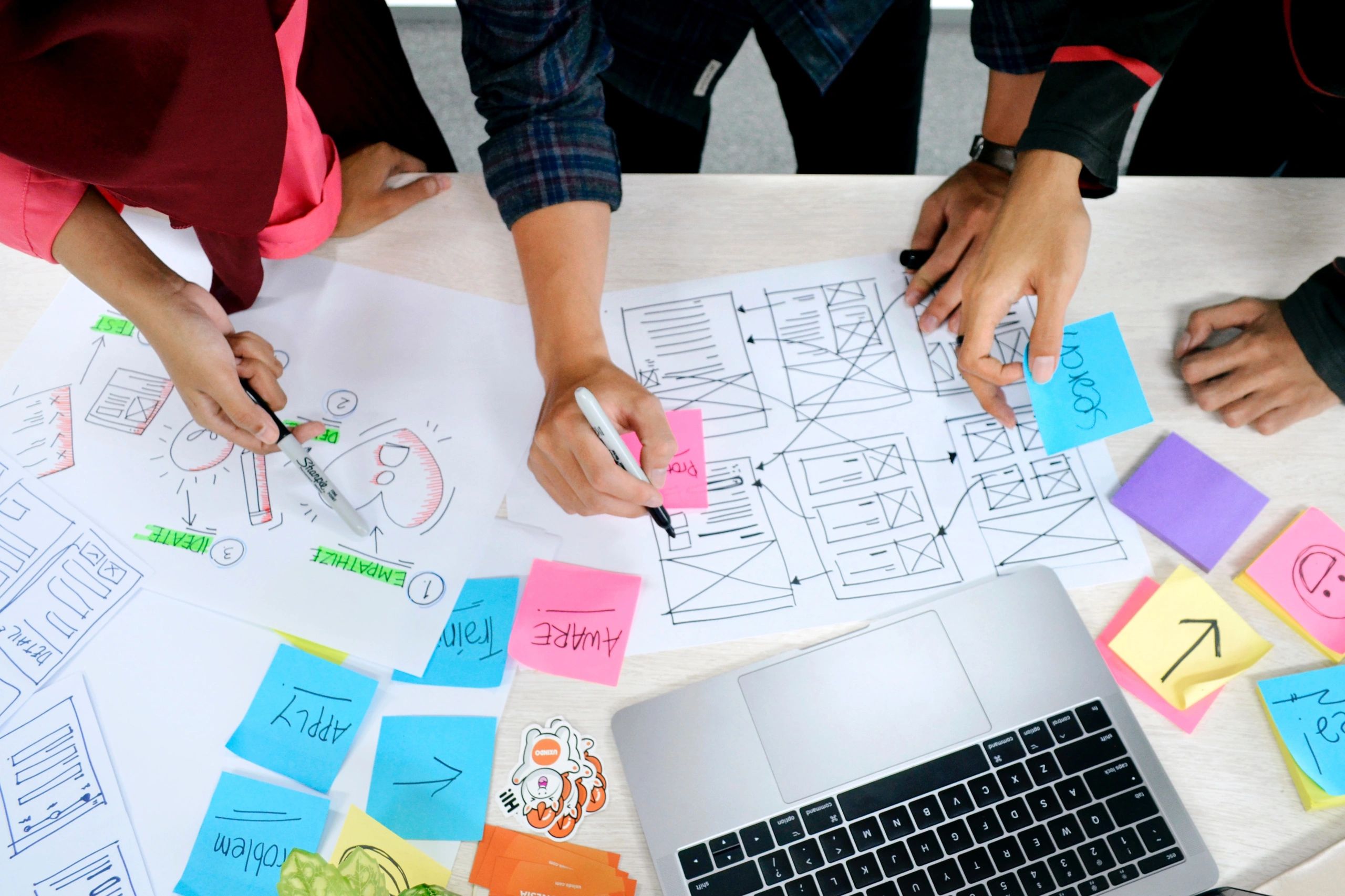
Start with a Model
Businesses use data to inform a product or service and discover and understand their customers. First, start with a hypothesis about the customer you want to serve or the job that needs to be done. This hypothesis, or model, comes from the reason you started your business. Then, you develop it further by asking questions that tell you what data you should seek. It’s helpful to brainstorm as many questions as possible and prioritize them. Table 1 shows the types of questions you might ask.

Once you have some questions, write down your best answers based on your knowledge. For example, let’s assume your team has developed a robot that can cultivate crops, discriminating between weeds and desirable plants, pulling the former, and appropriately tending the latter. This sentence gives you a starting description of the job to be done and some information about what’s desirable or not. Suppose your business goal is to solve a problem – capture in detail who has the problem and how they address it now. You may be guessing, but that’s ok – you’ll be using data to test your hypothesis next.
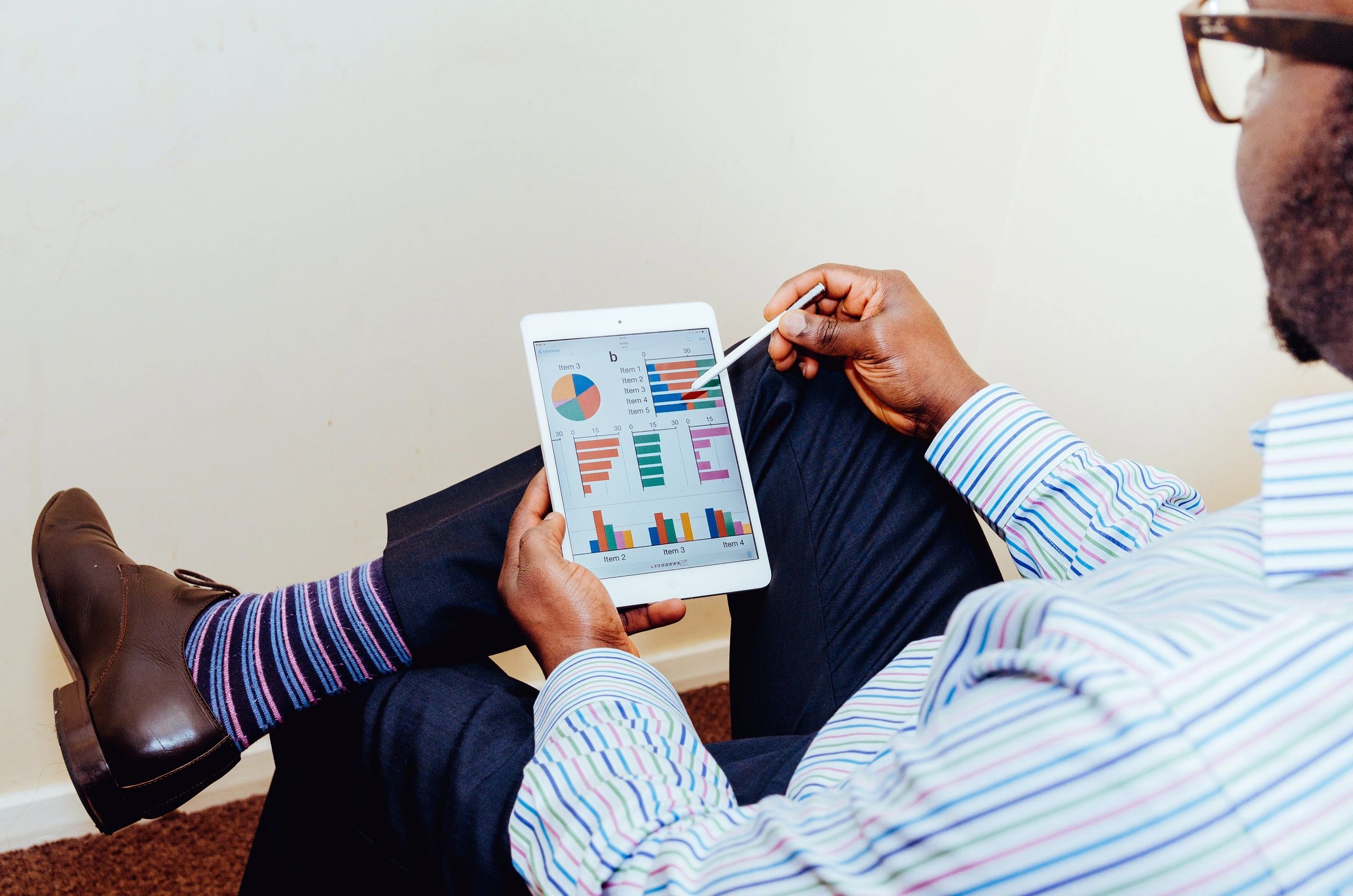
Data Sources
Next, you need data to test your model. There are three types of data sources which I’ve described below in the order of increasing effort and (potential) value. Your best approach is to take your highest priority question and validate your answer with the least effort. Document your search as you go and retain electronic copies of search paths and results – I’ve been frustrated in the past when I haven’t been able to re-trace my search steps for that perfect answer!
Curated databases
There are lines of business devoted to collecting and curating data, both generic and industry-specific. AtoZ Databases, Demographics Now, Morningstar, and IBISWorld are commonly available examples. I recommend you only use no-cost opportunities to access this information. If you don’t have a public library card, now is the time to get one (or several). Residents of my city, Spokane, WA, have access to multiple curated sources via the Spokane Business Library. More specialized sources may be accessible through the library system of your local college or university or your community service providers. For example, state employment security departments and city workforce boards frequently access labor market information through Burning Glass. Seek out network connections who can help you look for more sources. The US Government collects and distributes volumes of data which is readily accessible online. Examples include the EDA’s StatsAmerica and the Census Bureau’s Business Builder. A motivated and connected librarian is a best friend when finding unusual data. Please be thoughtful when you seek the help of others for data by preparing before your meeting, presenting no more than your top three questions, and thanking them for their support. I always try to ask them who else might be able to help me, which can accelerate my next step. With our example, you might use farm industry or government databases to discover which crops require a high level of human intervention and where the economics would support an automated solution.
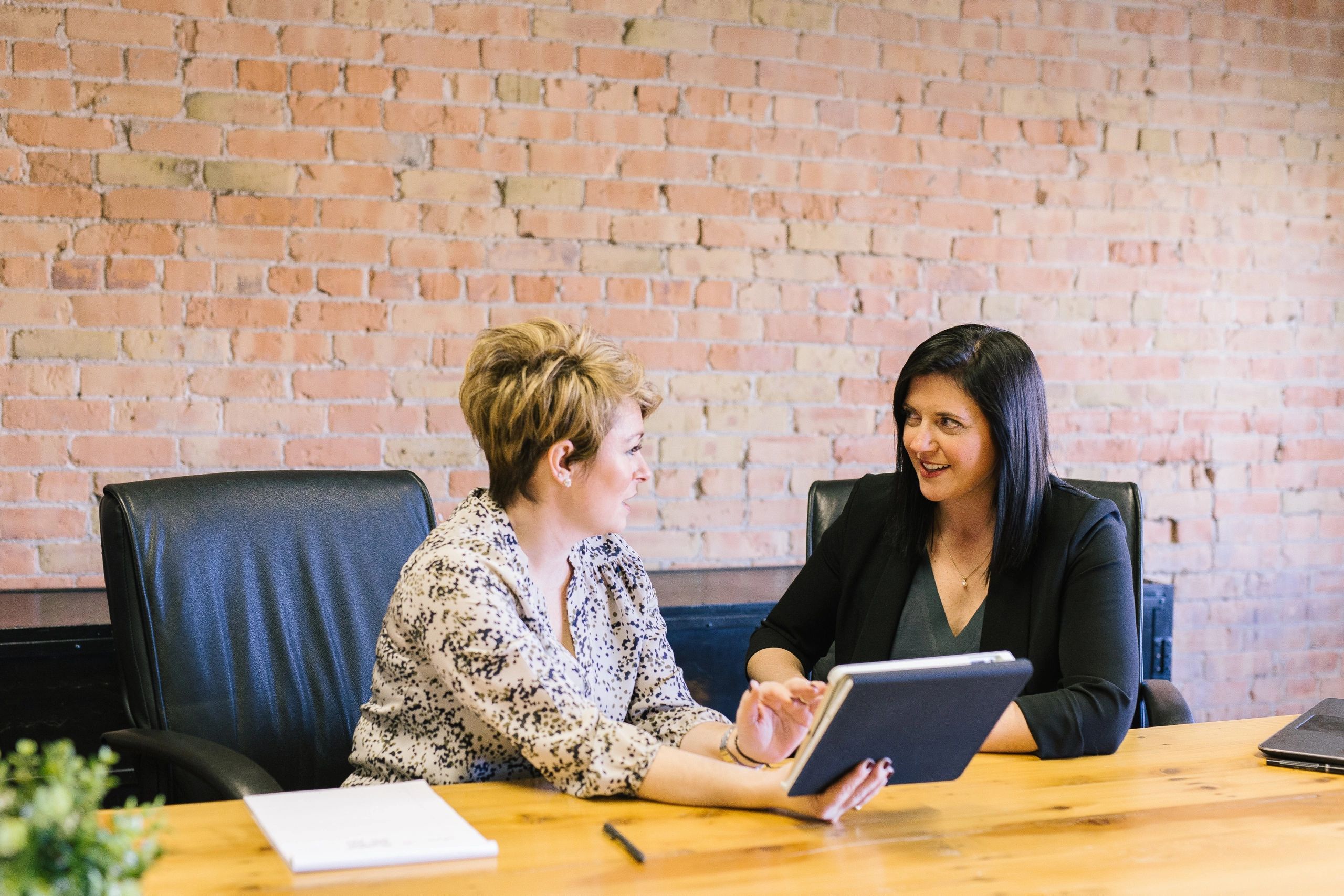
Curated databases
There are lines of business devoted to collecting and curating data, both generic and industry-specific. AtoZ Databases, Demographics Now, Morningstar, and IBISWorld are commonly available examples. I recommend you only use no-cost opportunities to access this information. If you don’t have a public library card, now is the time to get one (or several). Residents of my city, Spokane, WA, have access to multiple curated sources via the Spokane Business Library. More specialized sources may be accessible through the library system of your local college or university or your community service providers. For example, state employment security departments and city workforce boards frequently access labor market information through Burning Glass. Seek out network connections who can help you look for more sources. The US Government collects and distributes volumes of data which is readily accessible online. Examples include the EDA’s StatsAmerica and the Census Bureau’s Business Builder. A motivated and connected librarian is a best friend when finding unusual data. Please be thoughtful when you seek the help of others for data by preparing before your meeting, presenting no more than your top three questions, and thanking them for their support. I always try to ask them who else might be able to help me, which can accelerate my next step. With our example, you might use farm industry or government databases to discover which crops require a high level of human intervention and where the economics would support an automated solution.
Field Research
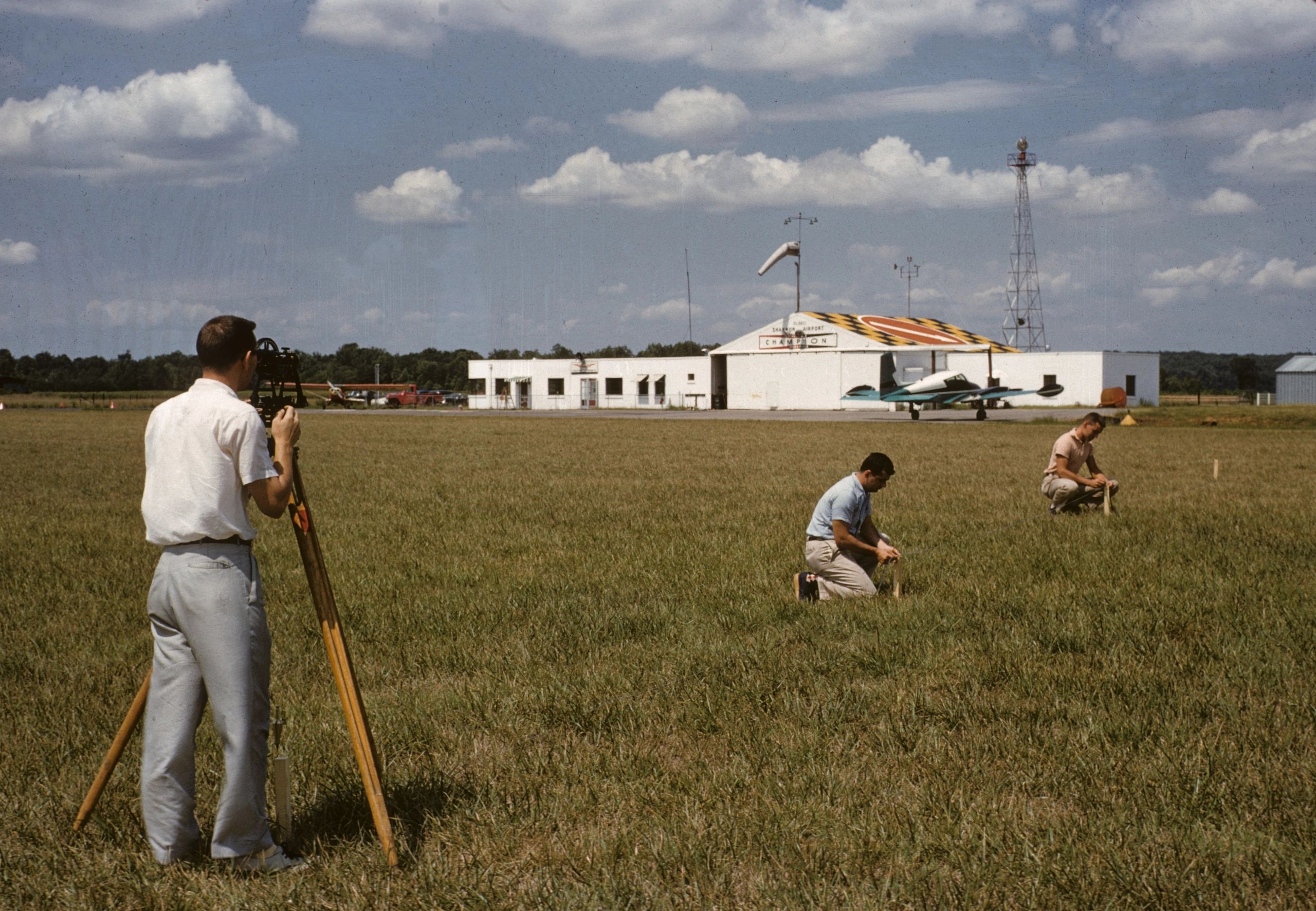
Field research requires the highest level of effort. Meetings with experts can overlap in this category. It also includes online funding campaigns, interviews, surveys (virtual or in real life), and other avenues of data collection and organization. These methods are time-consuming because you must think carefully about what questions to ask and how to ask them. The approach can be as simple as demonstrating your product and asking if the viewer would pay $X for it or more in-depth. A/B testing is a commonly used technique to test user preference on web pages, ads, or marketing emails. Founder-focused venture firm NfX shares a great list of potential customer questions in their tool “8 Playbooks for Building Viral Products.” If you have a group of customers to survey and know anyone who can help you use the Kano methods (1), you can use this approach to understand which attributes of your product or service are attractive or must have and which aren’t relevant. Field research returns the highest value when you have specific questions you need your customers to answer. With our cultivator robot, finding one or two farmers willing to pilot the device and provide usability and maintenance feedback could be a decisive step forward.
Wash, Rinse, and Repeat

Figure 1. Product or Customer Discovery is Cyclical.
You may have guessed that this process is continuous (Figure 1). Once you evaluate the assembled data, you should use it to improve your model. First, add new descriptors and context to elements with data support. Second, set aside those statements shown to be false. I recommend you keep versions of your records during the cycle – sometimes circumstances change to make previously flawed ideas more relevant. Third, document your sources so you can give proper credit and find them again. Lastly, start new hypotheses when you find new customers, markets, or products. The cycle is often more effective in short bursts, with the results being used immediately in strategy, business model, or operations planning.
Use, Not Misuse, of Data
I have three more tips to help you use, not misuse, your data. First, apply a beginner’s mind to the analysis. Your business model, product, or service may be entirely new. If so, the available data may make you think it can’t be successful. “It’s never been done before” is a hackneyed expression often discouraging innovative thinking. Instead, try adjusting your hypothesis and gathering more data, or use a different angle. As a result, you may define your space by the boundaries of what is known and adjacent. Second, recognize that we are all subject to cognitive bias and use tactics that help you minimize its impact (2). Identifying the different types of bias, permitting your team to discuss its effect, gathering information to measure its impact (for example, by using a pre-mortem), and monitoring the quality of decisions are all ways to address bias. Third, avoid the inclination to keep gathering data to “make a better decision,” an effect known as “analysis paralysis.” Time is a critical resource, and you will likely be better off with a field trial of something close enough rather than another round of data to refine your product or service. Just keep these tips in mind as you work. The discovery of product-market fit is a process, not a prescription.
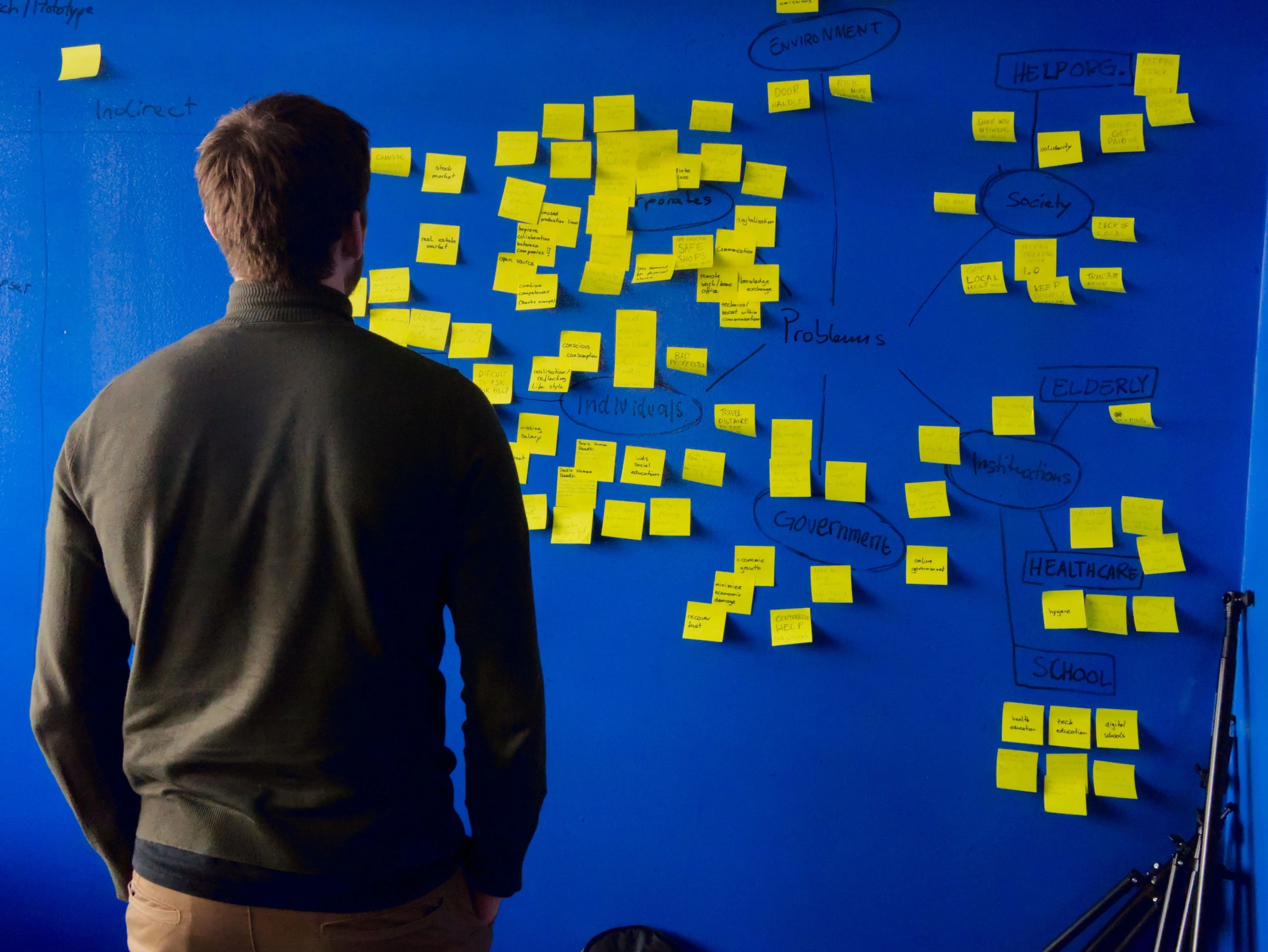
Those who know me will recognize my process approach to problem-solving in this guide. The variety of data available confounds us, and we don’t know where to start making sense of what we find in a quick search. Instead, we benefit by taking time to create a model from hypotheses, selecting a data source to answer a specific question, and incorporating the analysis into the model. Whether you call it requirements analysis, the scientific method, or problem-solving, a cyclical approach to customer and product discovery will give you a good start and a scalable system as you grow.
If you enjoyed this article and would like to read more by Katrina, sign up for her newsletter.
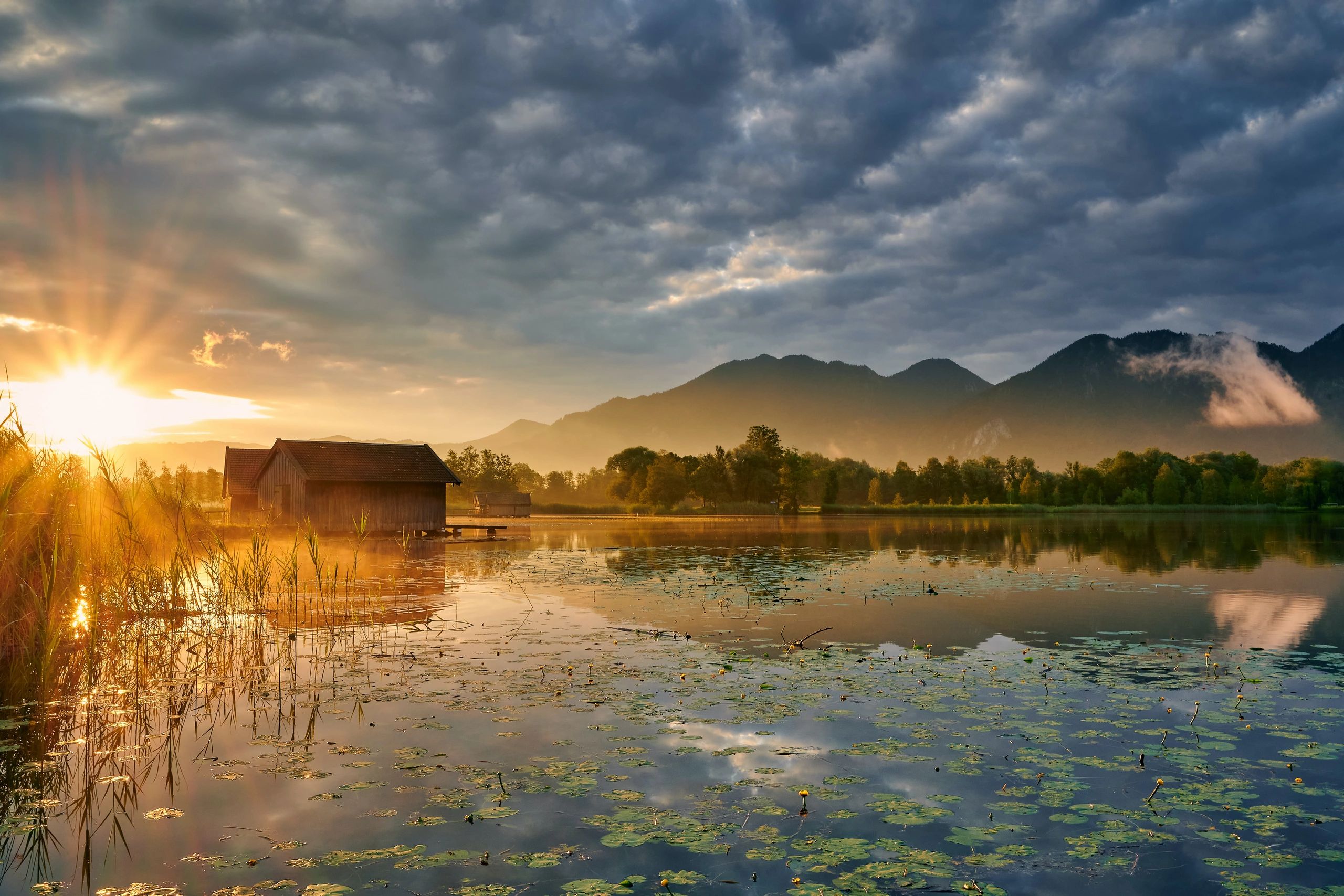